E-health: how data control is helping to revolutionize healthcare
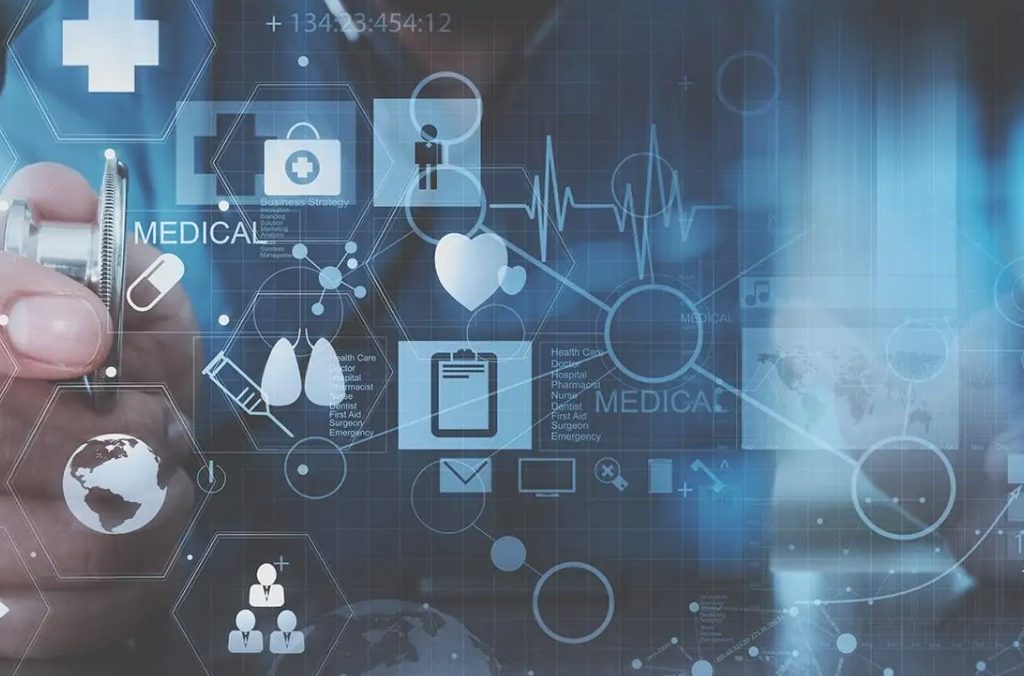
In a healthcare sector undergoing a revolution, data management has become the central issue for many players.
Indeed, it is thanks to data processing that medicine has been able to make its most spectacular advances in recent decades. The first sequencing of human DNA dates back only to 1977. It was not until 2003 that the first human genome sequencing project was completed.
In 20 years, data control and computational capabilities have exploded. Until a few years ago, the sequencing of the human genome was still very time-consuming and expensive. The exponential progress of bioinformatics has made it possible to change scale and open the door to the advent of personalized medicine.
What are the major health issues that data management addresses? What are the challenges faced by the players in the ecosystem and how does the Loamics solution contribute to meeting them?
The context: from standardized to personalized medicine
A revolution in progress
The healthcare sector is currently undergoing a real revolution: the transition from standardized to personalized medicine.
Until now, medicine has been largely standardized. Patients with the same pathology all receive the same treatment. Then, according to their reaction to the treatment, they are divided into 3 groups:
- The first group reacts positively to the treatment. The pathology resolves.
- For the second group, the treatment has no effect.
- Finally, for the last group of patients, the treatment has negative effects and the health condition worsens.
In this conception of medicine, we consider the pathology but without taking into account the environment in which it develops, i.e. the individual characteristics of the patient.
Today, the progress made in sequencing the patient's genome makes it possible to identify the genes that predispose to a disease, and even to establish the genetic profile of cancer cells. By formulating a diagnosis based on several biomarkers, patients can then be segmented into several groups, each of which will receive a treatment adapted to their diagnosis.
Since the treatment is adapted to the specificities of the patient, the chances of recovery are greater. This is what we call personalized medicine. In this new logic, doctors no longer treat a pathology but contextualize the treatment according to the multiple data they have been able to collect and analyze.
Data at the heart of personalized medicine
Personalized medicine relies on the ability of e-health players to collect, centralize, process and analyze biological data from various sources. In this perspective, genome and metagenome sequencing is becoming a major discipline.
Indeed, personalizing diagnoses and treatments requires the integration of large-scale "multi-omics":
- Genomics
- Transcriptomics
- Proteomics
- Metabolomics
In addition to genome sequencing, personalized medicine requires the processing of massive data on other variables of the ecosystem. Knowledge of the microbiome system is particularly crucial because it directly impacts the effectiveness of treatment.
In the human body, 10 to 16 bacteria coexist to create a stable system. If we want to know precisely their components and their interactions, the volumes of data are immense. As a result, biotech companies need large computing capacities.
Personalized medicine therefore implies high-throughput sequencing techniques allowing a better description of microbial diversity and potential biological functions by the whole community.
E-health and data: what are the challenges for the players?
The exponential increase in health data
The deployment of new technologies since the 2000s has led to an exponential increase in the volume of health data. On a global scale, the total volume of e-health data doubles every 73 days!
The Internet, social networks and cloud computing have facilitated the generation and sharing of information. The development of connected objects favors the generation of health data collected directly from the user. The deployment of 5G will further amplify this data massification.
Practitioners and healthcare institutions also generate large data sets: patient results, clinical trial data, genetic data, bioclinical data, pathological data, pharmacy prescriptions, laboratory results, etc.
What uses for health data?
The massive generation of health data is a reality.
One of the challenges of e-health is to acquire a real mastery of the data in order to make use of it in ways that are beneficial to doctors and the population:
- Diagnostic assistance
- Personalized medicine
- Shared knowledge bases
There are many stakeholders interested in e-health: doctors and healthcare institutions, patients, local authorities (in the context of health monitoring and public health policies), research institutions, biotech companies, etc.
Challenges related to data
Data opens up new opportunities for innovative use cases in healthcare. But the mass of heterogeneous data to be managed also raises new challenges:
- Data storage: collecting and centralizing health data on a large scale requires substantial storage capacity. It is necessary to be able to store and integrate any type of data needed.
- Computing time: the data collected is heterogeneous. As it is, it cannot immediately be used for analysis. The second challenge is to homogenize the data to make it usable in use cases.
For example, until a few years ago, genome sequencing was very time-consuming and a sequencing run cost billions of euros. Today, 4 to 10 genomes can be sequenced in one day for a unit cost of around €1000. This is simply because the tools exist to collect the data, homogenize it, control its quality and automate the machine learning and artificial intelligence workflows.
A large part of the data "cleaning" work that the data scientist had to do is now automated. They can now focus on high value-added tasks such as developing new algorithms and interpreting the data.
Data collection, data quality control, data standardization and workflows become "back-end tasks". They are done in much shorter timeframes and at lower cost. The effects are multiple: accelerate the discovery and description of a new pathology or accelerate the marketing of a new treatment ...
How does the Loamics solution meet the challenges of e-health actors?
Loamics, a horizontal solution for optimal data control
Loamics is a horizontal solution that supports healthcare actors in their desire to better master data in order to develop data-based business applications.
The solution is based on 3 main components:
- Data Collect: the solution collects massive and heterogeneous data, regardless of the type of data and its source. For example, for laboratories working on health, the data can be biomarkers, microbiota data or massive sequencing data.
- Data Lake: Loamics centralizes the data in a single data warehouse, but above all the solution makes the data usable. It homogenizes the data to make it usable for decision making or for the development of business applications.
- Algorithms: Loamics makes it easy to automate machine learning or artificial intelligence algorithm scenarios on previously cleaned and reliable data.
Thanks to these three components, the solution considerably accelerates the digital transformation of the biotech sector. Previously, data control and protocol validation were very time-consuming. This part is now automated to quickly isolate a good quality sample.
Ultimately, Loamics addresses the triple challenge of data storage, homogenization and computation time.
A data architecture that opens the way to diversified uses
Loamics frees teams from the work of data cleansing and allows them to focus on the interpretation of analyses and the design of new solutions.
The use cases are multiple:
- Customized analyses in partnership with ecosystem players: the Loamics software solution supports innovation, leading to the filing of combined patents, for example with biotech companies.
- Analysis of existing public data: Loamics enables the networking of health data for better international collaboration between actors. We are developing a networked health database in the form of a DataMart.
- Diagnostic assistance: the opening of medical data in France encourages the networking of information. With Loamics, it is possible to import textual data from medical files and process them with an AI algorithm to extract medical rules and facilitate diagnosis by comparison with an ontology.
The explosion of data is about to revolutionize medicine. But for this to happen, professionals in the sector must gain control of their data.
Data storage, homogenization, calculation time, the Loamics solution contributes to health innovations by providing actors with an architecture to automate part of the data path and accelerate the development of business applications.