How to ensure real data governance to optimize the use of data?
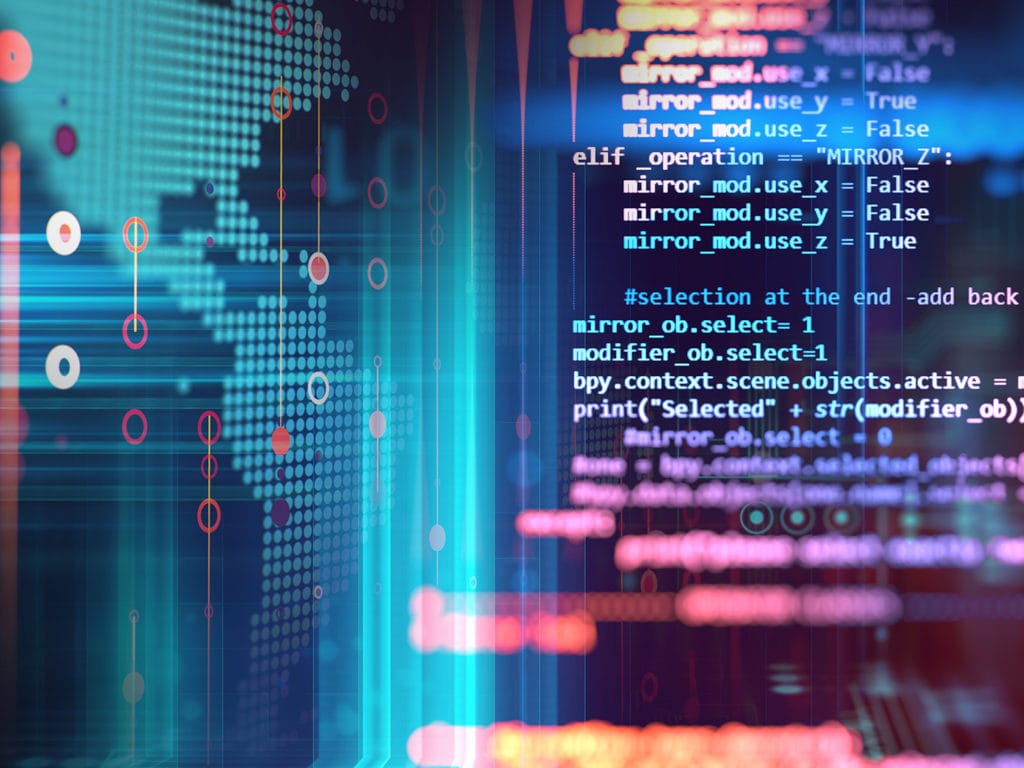
Companies, local authorities and organizations in general now generate huge amounts of data.
The goal is to make this data available, exposable and exploitable to feed business uses. Whether it's to automate a process or to support decision-making, data plays a central role.
But first, raw data must be transformed into usable data for the business.
Data processing is at the heart of all data-driven processes. It is this processing that enables data to be cross-referenced and standardized to make it not only usable, but also reliable and relevant.
However, to ensure business uses, not all data is useful to everyone.
How can we ensure that everyone has easy access to the data that is necessary and sufficient for their missions? How can we ensure the availability, usability, integrity and security of the data used in an enterprise?
The answer lies in data governance.
In this article, we take stock of the challenges of Data Governance. And we explain how the Loamics infrastructure, with its data approach, allows to ensure a real and operational data governance.
What are the challenges of data governance?
Responding to a situation of data massification
In organizations, the amount of data available is greater than ever. Let's take the example of a company. Each department generates an unprecedented amount of data: marketing and sales data, accounting and financial data, personnel management, operations, purchasing, etc.
The increase in the amount of data is one of the consequences of the digital transformation. From now on, data is the fuel that allows to digitize and automate certain processes or to support decision making. This increase is also made possible by the democratization of new technologies that facilitate the collection of data.
In the era of Big Data, the question is not so much to collect data. It is more about knowing how to make the most of the data collected.
And between data collection and business uses, data processing is an essential link. At this stage, it is a question of standardizing and homogenizing data to make it accessible, reliable, usable, profitable and secure.
Data governance presides over this "regulation" of data. It is at the crossroads of several issues: technical, regulatory, organizational and economic.
Overcoming the pitfalls of data processing
The processing of massive and heterogeneous data presents several pitfalls:
- Duplicate data: several data sources use the same data but in a different for
- The varied nature of the data linked to the various functional domains: some data are attached to several functional domains of the company
- Non-homogeneous reference data (nomenclature) specific to each department: each department has developed specific rules for processing the data that concerns it
- Different time scales, measurements and units
ETL processes are designed to take raw data, extract the information needed for analysis, transform it into a format that meets operational needs and store it in a data warehouse.
However, traditional ETLs work according to a "system and process" approach. The business rules of each department therefore determine how the data is processed. And, therefore, the pitfalls of data processing persist.
A true data approach consists in basing itself not on theory but on facts, by decoupling ETLs from use cases. In this approach, which is the one used by Loamics, the ETLs are linked to the data catalog, thus allowing real and efficient interoperability.
The benefits of a real and operational Data Governance
Data governance is a key success factor of the data approach in organizations. Its benefits are multiple.
First of all, it allows you to work with data in an optimal way. It contributes to making data available, exposable and exploitable for business uses, in particular for non-IT business profiles.
It also facilitates the "breakdown" of necessary and sufficient data for end users in their uses. With effective data governance, you can create "self-service data" and manage access rights for different categories of users.
Data Governance helps to reinforce the actual quality level of the data, but also its security. It allows you to be free from the origin of the data, its source, its heterogeneity and its complexity and to facilitate analysis and business uses thanks to relevant, reliable and secure data.
How to ensure an optimal Data Governance?
Treat primary data and metadata
To work efficiently on data, you must be able to take into account all the values of the data:
- The primary data: for example, a temperature
- The metadata: this is the data that allows to contextualize the primary data. In the case of a temperature, the metadata can be a unit (degrees Celsius or Fahrenheit), a location, a date and time, the position of the message (for example, the 14th of 24)
All these data values exist but they are not all equally useful to feed the business-uses. Depending on the use, only certain values will be necessary.
Data governance consists precisely in selecting the values that we want to send and only those. The business profiles then receive only the data that is necessary and sufficient to perform their task. For example, you can choose to send only the secondary values without the primary data.
Set up a Data Catalog
The Data Catalog is the perfect tool for defining data, as well as its structure, source, quality and use. It is also a collaborative tool that guarantees the proper use of the data.
A Data Catalog can be compared to an inventory, a dictionary of a company's data. Intelligent and practical, it facilitates data management by defining and organizing all data values on the same level, from the primary data to all metadata.
You thus obtain a set of standardized, reliable and easily actionable data to derive business value.
The data catalog addresses several issues raised by data:
- Where to store all the accumulated data?
- How to build intelligible data pools?
- How to avoid duplication between different databases?
- How to structure all the information to meet the needs of all the company's businesses?
In concrete terms, to set up a data catalog, you must first make an inventory of all the values (primary data and metadata), then tag all these values. This is the role of the data steward. Thanks to the tags, you will be able to establish rules and access rights and define which values should be released and to whom.
How Loamics optimizes data governance
The Loamics solution meets the challenges of data governance because it is based on a data approach and not on a systemic approach like other solutions. Instead of starting from processes and working on data silos, sometimes duplicated from one department to another, Loamics offers a bottom-up approach based on facts.
On the front end, the infrastructure is able to collect all the data and metadata passing through the organization, inventory it, tag it and make it available to business users within a data catalog.
Data virtualization is another asset of Loamics. It enables organizations to access data from disparate sources and provide unified visibility into data faster, at lower cost, and using fewer resources than traditional data integration approaches.
Data virtualization offers two key benefits:
- Reducing delivery times compared to extract, transform and load (ETL) processes.
- Enable organizations to effectively integrate and manage their data where it resides, without replication, and enable technical and non-technical users to quickly answer key business questions using a data-driven approach.
Want to make data more available, reliable, and accessible to business users?
With its data approach, Loamics infrastructure does not prejudge the uses that will be made of the data. It facilitates the governance of data for business uses in an open approach that favors the exploitation of data, the control of costs and processing times as well as the creation of specific datasets.