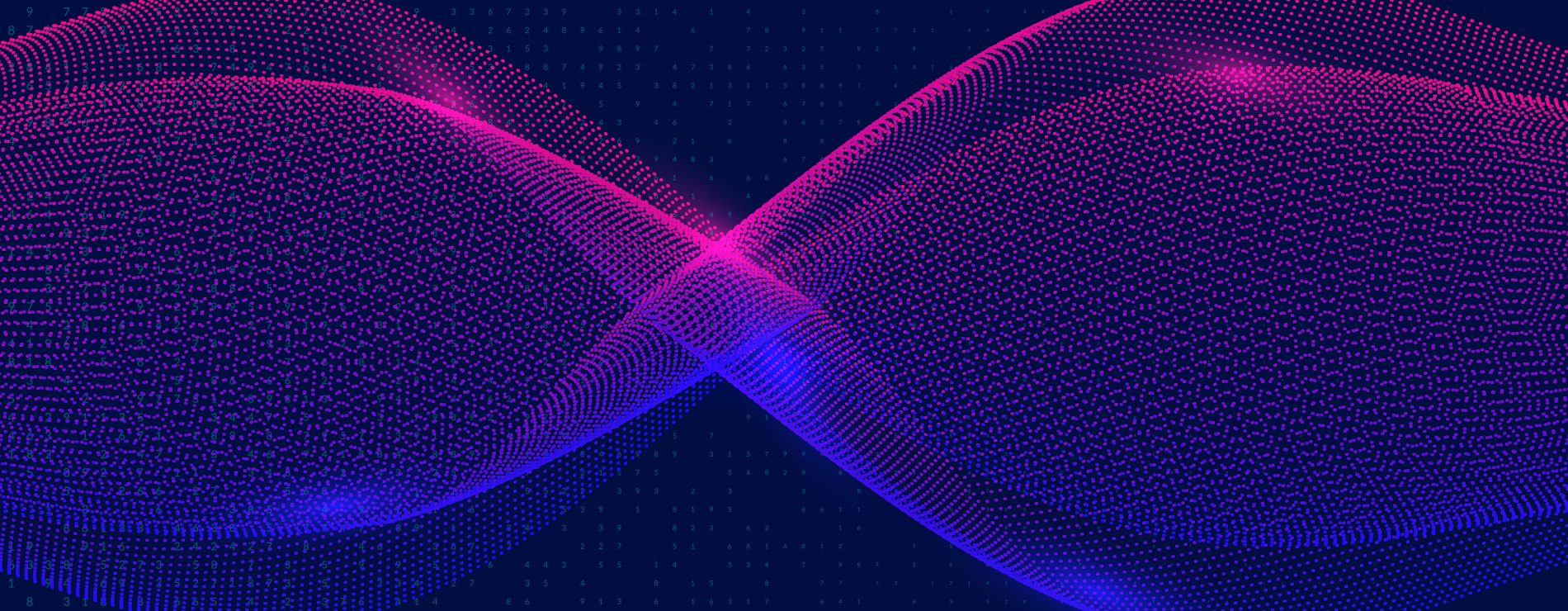
AlgoEngine
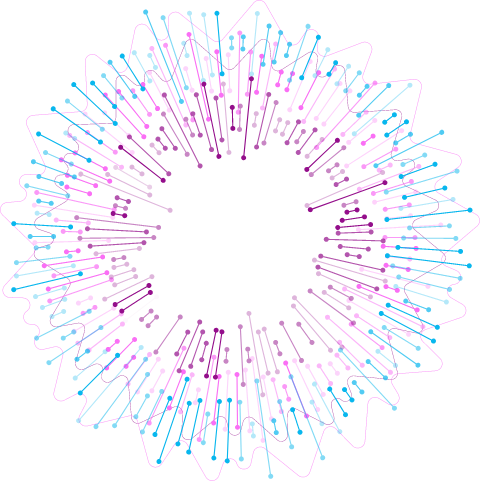
What is a data engineering?
Data engineering focuses on the elaboration and structuring of data flows to enable optimal exploitation. This step in the data processing process is crucial in view of the multiplication of data flows and the quantity of data. Gartner, the leading consulting firm in the field, defines data engineering as follows:
“Data engineering is the discipline of making the right data accessible and available to different types of data consumers”. It includes data scientists, business analysts, data analysts and many others across the enterprise.

To organize, structure and select data
The objective of data engineering is to select, sort and arrange data in a way that guarantees its quality and relevance. Data engineering is therefore an essential complement to data science.
The two disciplines, which were once confused, are now distinct from each other. Without data engineering, companies can quickly suffocate under the weight of useless data. Do you remember the expression "finding a needle in a haystack"?
This perfectly illustrates one of the primary functions of data engineering. The objective of the data engineer is to identify, access and use relevant data.

Data pipelines and data science models
The very basis of data engineering is the creation of data pipelines.
Like other kinds of engineers, data engineers imagine and build structures. Data engineering must allow for scalability as well as optimal security.
Another aspect of data engineering is the production of data science models. In recent years, many tools have emerged to facilitate this aspect of the work.
This is notably the case of the LOAMICS-Suite Totale and its AlgoEngine module.
This organizational work is essential. The percentage of data science projects that make it to production is around 87%. One of the major reasons for this low success rate is that data exists in different forms, in different units with different security or privacy protocols. So, the data needs to be collected and cleaned up to allow it to be used. Moreover, the collection, processing, and analysis of data in real time is crucial for the development of machine learning models and artificial intelligence algorithms. Indeed, to ensure proper functioning, the quality of the data, especially the training data, makes a real difference.
Connect algorithms to your data
Machine Learning is a set of techniques used by Data Scientists that has been widely talked about in the last few years.
Because its applications are varied and very promising.
AlgoEngine provides you with all the algorithms that allow you to connect your data to these revolutionary algorithms.
It is the one that connects the data in the data lake to the visualization applications, dashboards, and predictive analytics you may want to develop.
Once your data scientist has collected, cleaned, and mined the data, he or she can create a Machine Learning model. This model connects the data it receives as input to the results it gets as output. Instead of performing calculations using traditional algorithms, it establishes a statistical link between the new data to produce new results. This connection between data, via ML algorithms, is called learning. In fact, your models can be re-trained on newer data to provide even more accurate and relevant predictions. It is all this magic on data that AlgoEngine can accomplish. The module is also capable of deploying traditional algorithms that have proven successful in the past.
Discover our other software

AlgoEngine is part of the LOAMICS-Suite Totale and is in fact its third and final module. This last link in the chain, the ultimate step in the data processing pipeline, relies on two other software programs. DataCollect collects data from any source, in any format and in real time. Then there's DataLake, which, as the name suggests, is the storage software that works in synergy with your cloud instance to provide secure access to your enterprise data.

Our software is constantly evolving in terms of technology. We are currently strengthening our cloud virtualization abilities, increasing security of customer data, and scaling up our AI capabilities. Our software provides simplified access to data, making it easier and faster to create value. The security of the company's data assets allows for tighter governance.

This simplified access to data also enhances team collaboration and information sharing. All companies using LOAMICS AlgoEngine can benefit from the ease of data processing. The origin and the conditions of collection have no impact on the use of the data. The interoperability between tools and uses allows an unlimited number of deployment types.
Our other software
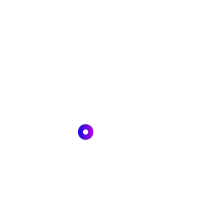
01 DataCollect
Collect and ingest raw data in real time (regardless of the volume, sources or format), to be very simply transformed into homogeneous, efficient and valuable enriched data ready for data visualization and first levels of analysis.
See more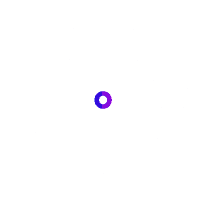
02 DataLake
Provide access to all metadata (contextual data) in a key value system. Store and access proprietary data in a single, elastic, scalable system hosted within the organization. The data is ready to be exposed without the need to replicate. This data is prepared for analysis and artificial intelligence.
See more