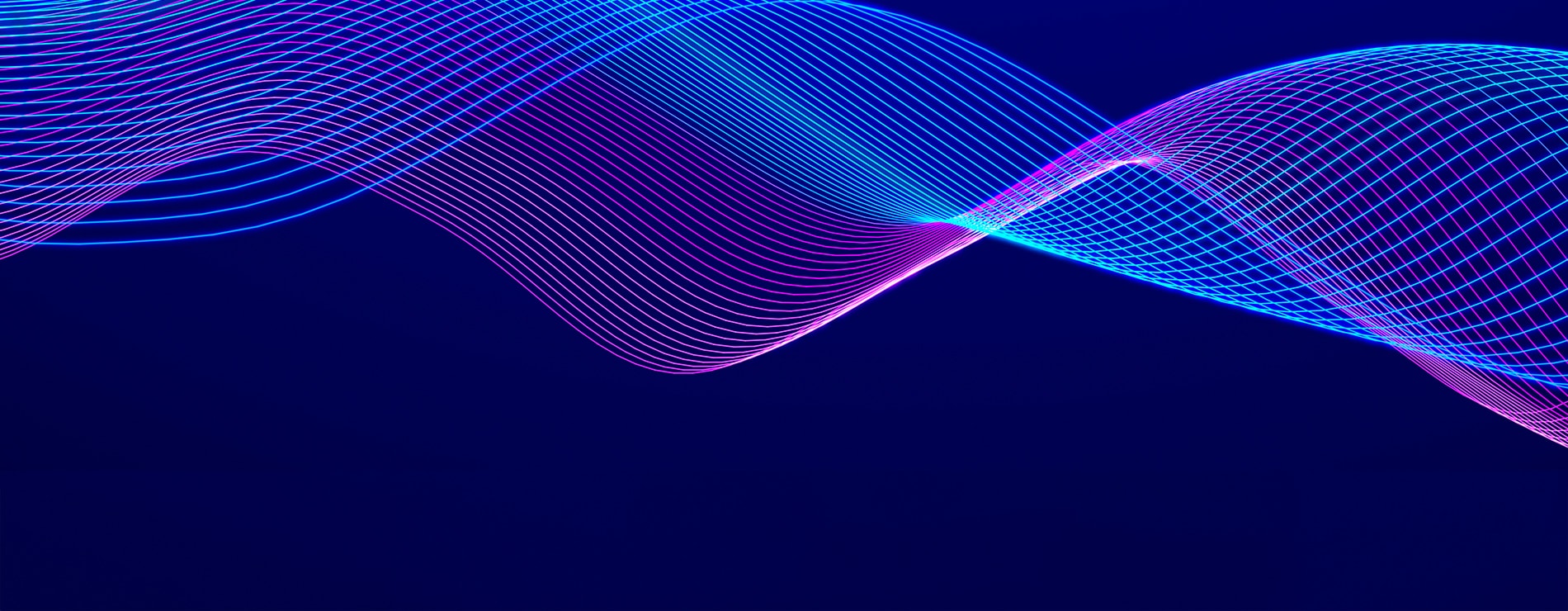
Augmented analytics & business intelligence (BI)
What is augmented analytics ?
The simplest definition of augmented analytics is the use of machine learning (ML) and natural language processing (NLP) to better analyse data. Data is extracted, filtered and analysed to find AI patterns and relevant informations, which will generate automatic and rapid responses that can be communicated without the intervention of a data scientist or an analyst.
This method makes it possible to industrialise the processing of data with a view to its exploitation. The notion of augmented analytics was conceptualised by the research firm Gartner in 2017, in their report "Emerging technologies: hype cycle".
Indeed, the information provided by data within a company can be quite vague. For example, if the data show that your revenues are declining, knowing this will not help. It is then necessary to identify the source of the problem as well as other malfunctions that may be related. Among other things, uninterpreted data is superfluous.
Now, augmented analytics allows a deeper analysis of what this data really mean.
It analyse all the resources to extract the relevant informations.
These tasks traditionally performed by analysts and data scientists are now automated by using machine learning techniques, and in particular natural language processing (NLP), to optimise the analysis of data at every stage of the data life cycle, from its preparation and formatting to the resulting indicators.
These methods thus constitute a new stage in the democratisation of business intelligence. Like interactive self-service dashboards such as Microsoft Power BI which allows users to ask their questions directly to the BI application via the translation of graphical queries into SQL queries by the machine, the software will then answer them by generating personalised indicators explained in natural language and in graphs, taking into account the needs and business context of the employee.
To answer the queries, the results of the augmented analysis are displayed in a relevant way with visual representations. These representations help users to better interpret the data, but also to initiate strategies to improve the company’s revenue.
The role of machine learning in augmented analytics
As we have seen above, augmented analytics relies on ML techniques and in particular NLP to optimise data analysis. So machine learning is the main actor in this method, but various ML techniques are used in different cases, including :
Loamics AutoML based on historical data & multiple statistic model allows to find automatically a KPIs Insight. The use of machine learning in augmented analytics simplifies the process of creating and simulating trends. Loamics AutoML program includes several functions: clustering, classification, prediction …
Augmented data preparation allows you to integrate lists of reliable data more quickly . The Artificial Intelligence program of Loamics « Data Cleaning » finds missing and error data, corrects and fill with the new good data. The quality control of the data can be set up quickly as well as profiling, tagging and various annotations on the data. Tools such as Microsoft Power BI allow, regardless of the data source, to be accessed quickly, with a simple user interface.
Another characteristic of augmented analytics platforms is the use of Natural language processing which is a linguistic field combining computer science and artificial intelligence which aims to create natural language processing tools, Natural Language Querying which uses the same techniques as NLP but this time to query information using natural language which allows the platforms to be used via a search engine, and finally Natural Language Generation tools to return the results of the search engine.
Some platforms allow the creation of simple Dashboards and visualisations for business users. These platforms allow the creation of charts in an automated way. For example, some tools, via dedicated modules, allow you to query as you would in google via NLQ techniques to generate visualisations automatically.
These are just a few examples of how can different machine learning techniques can be integrated in the main augmented analytics platforms. But there are many more, such as automatic report generation, trend prediction etc.
What are the benefits of augmented analytics ?
“Augmented analytics will fundamentally change the user experience for analytics,” says Rita Sallam of Gartner. Combined with human curiosity, artificial intelligence makes augmented analytics a very effective way to quickly obtain the information contained in data.
By offering to transcribe user requests into SQL queries, the various augmented analytics tools will allow users to simply formulate their request and receive an immediately understandable and relevant response. This helps to improve productivity and make the best business decisions for a company for several reasons:
- Faster data analysis
- Deeper insights
- Exponential confidence
- Better interpreted data
- The democratisation of data
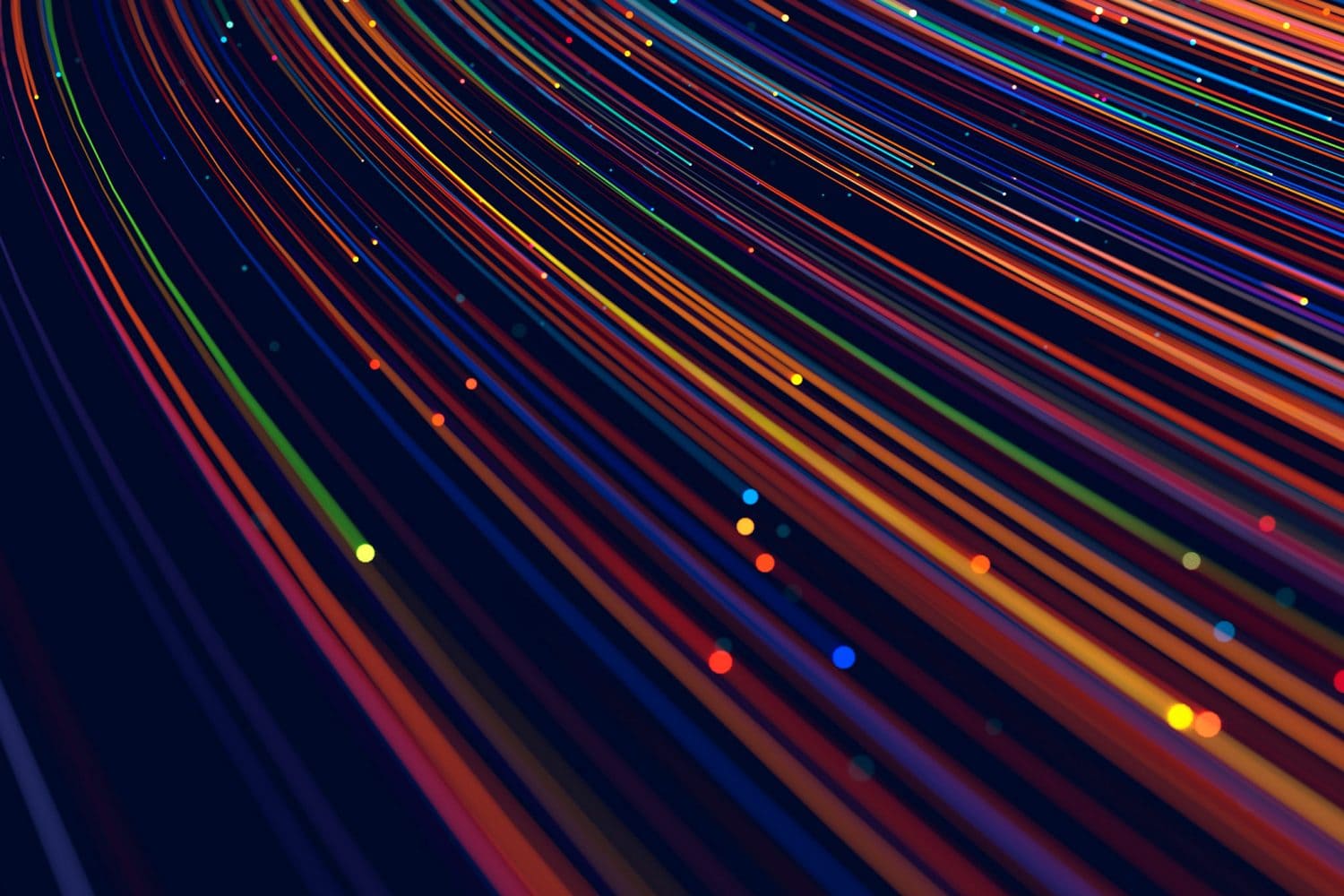
When combined, data science and artificial intelligence will ensure that data is prepared faster. This also means faster visualisation, faster search results and therefore higher productivity.
Thanks to the algorithms used in augmented analytics, it is easier to combine different data sources, and to clean them up.
Simply drag and drop on the visual interface to generate charts, maps, KPI objects and other data visualization modes according to your preferences.
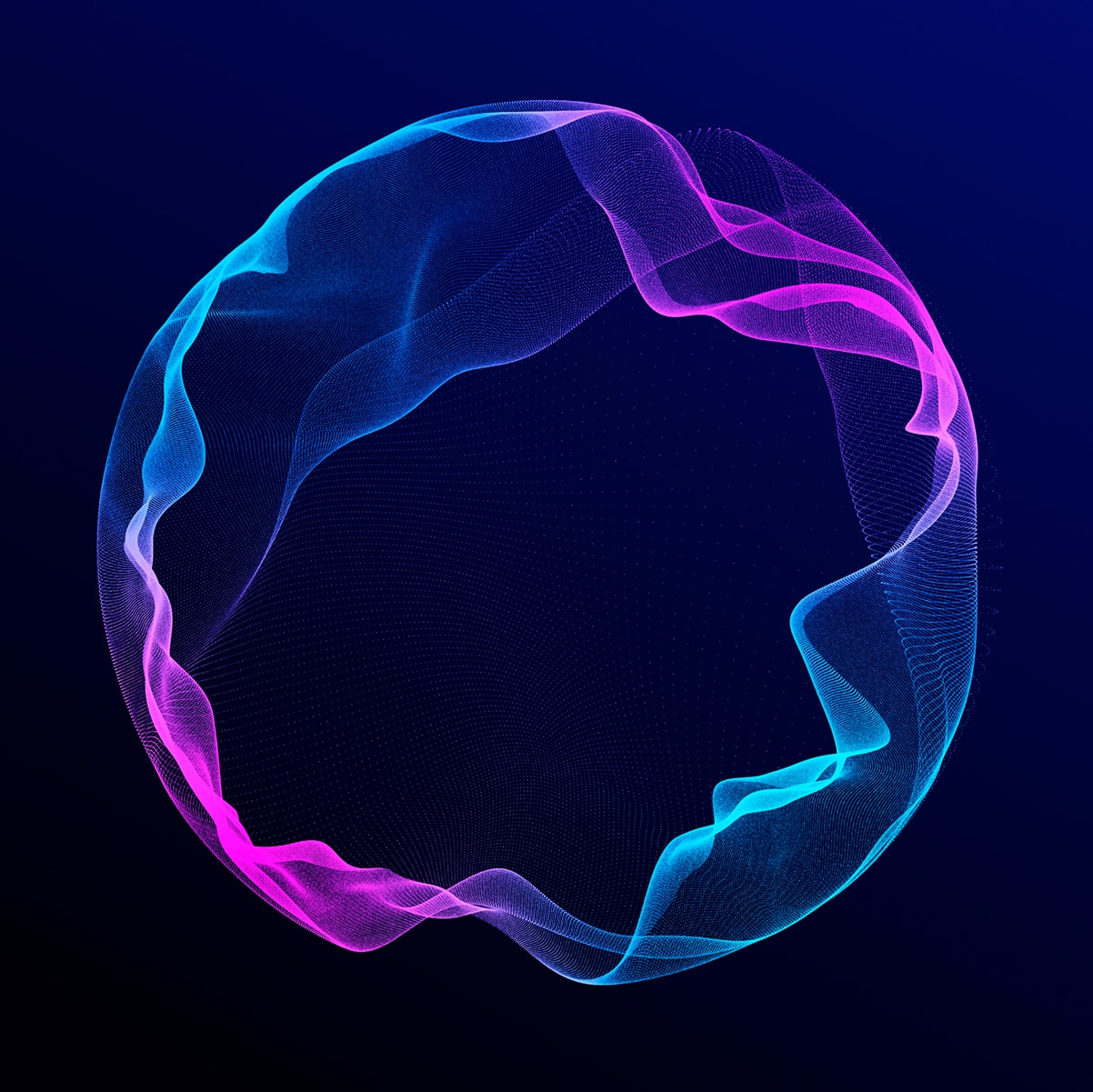
Microsoft x Loamics
Created in 2020 by data specialists, Loamics, has developed a PaaS technological infrastructure that ensures fully automated data processing, which enhances the value of the data and makes it immediately available.
This PaaS infrastructure is available in the Microsoft Azure Marketplace through a partnership between Loamics and Microsoft which believed in this project very early on by giving Loamics access to the partner network, and now the platform can connect directly and automatically to all Microsoft services and is capable of processing massive heterogeneous data continuously or in Real time in a fully automated and industrialised manner.
Loamics, as a partner of the Microsoft Partner Network, has direct access to companies, organisations and administrations throughout the world while benefiting from local support by Microsoft teams, and Loamics customers can now take advantage of the productive and trusted Azure cloud platform, with streamlined deployment and management. This platform enables players in all sectors of activity to have real “data-driven” control and decision-making based on intelligent, reliable and secure cross-referencing of data.
Loamics also took part with its partner Microsoft in the Environmental Start-up Accelerator initiative, a 6-month acceleration programme for 7 to 10 European start-ups working to reduce and offset carbon emissions.
Our latest augmented analytics use cases
Augmented analysis can be found in various fields such as e-commerce, marketing, supply chain or management of communication tools. It facilitates decision making and is relevant in many situations. Financial services are, without a doubt, one of the most promising areas for augmented analytics.
In particular, augmented analytics solutions can save financial analysts valuable time in the face of the complexity of their ever-changing business environment, both in terms of regulations and market conditions. However, we at Loamics, design and implement solutions that are usage-independent and can work with all types of data, and we’re going to show you two software solutions that we have developed for two use cases :
DataCollect
The goal of datacollect is to collect and ingest raw data in Real time (regardless of its volume, source or format). The data is transformed into a homogenous, efficient and valuable enriched output for data visualization and first level analysis.
Loamics and MydataModels software
Loamics and MydataModels, two French deep tech start-ups, have teamed up to maximise the value and accessibility of data. Together, they have created an augmented analytics solution allowing rapid analysis of large volumes of data in order to make strategic decisions.
Combining Loamics’ powerful infrastructure – which automates and industrialises data preparation – with the intelligence of MydataModels’ AI and predictive modelling platform offers a unique and unparalleled solution in the Big data market, rivalling expensive solutions from mainly American competitors.